The AI Agent operating system has raised $56 million in funding.
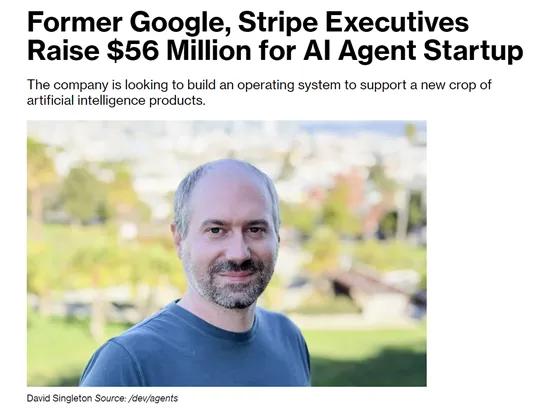
AI Agent startup platform /dev/agents has announced $56 million in seed funding. The round was led by Index Ventures, CapitalG, Andrej Karpathy, co-founder of OpenAI, and Alexandr Wang, CEO of Scale AI. The funding is also one of the highest seed rounds in the global AI Agent space, highlighting the technical prowess and commercial potential of /dev/agents.
The AI Agent is a high-level technical evolution of current large models, used to process information and automatically perform tasks without human intervention. It is not just like a chatbot that answers questions and summarizes documents, but also integrates the ability to use tools and operate autonomously, using human-like reasoning to make decisions. For example, it can send emails, write and send tweets, send reports and analyze data, etc.
Modern AI is changing the way people use everyday software. AI Agents can make PCs help people quickly execute various complex, repetitive workflows like teammates and colleagues.
According to /dev/agents, the current pattern of building AI applications and AI Agents is occurring in a fragmented environment of different operating systems and settings, which hinders the application. To achieve the vision of getting AI Agents into more people’s hands, it means introducing new developer tools, creating new user interface patterns, and rethinking application design around AI Agents themselves.
In the Talker-Reasoner proposed by Google DeepMind researchers, the System1/2 is cleverly integrated into the AI Agent. The core idea of the Talker-Reasoner architecture is to divide the AI Agent into two independent modules: Talker and Reasoner. These two modules correspond to human System 1 and System 2, respectively, and each has its own task and function.
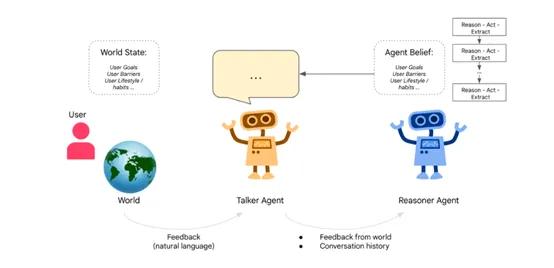
In the Talker module, the AI Agent is designed to understand and process natural language, generate coherent and natural conversations. This requires the AI Agent to have strong language understanding and generation capabilities, as well as sensitivity to context. The Talker module needs to be able to quickly retrieve relevant information from memory to support its conversation generation.
This memory can be a conversation history, user preferences, or other relevant information. The design of the Talker module enables it to simulate human intuition, allowing for reasonable responses even in cases where information is incomplete.
Unlike the fast-response of the Talker module, the Reasoner module is designed with a focus on deep thinking and complex problem-solving. The Reasoner module needs to be able to perform multi-step reasoning, which may involve analyzing and processing large amounts of information. It needs to call various tools and databases to obtain external knowledge to support its reasoning process.
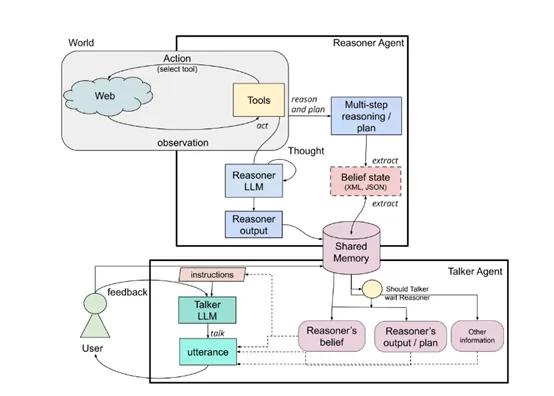
The Reasoner module also needs to be able to form and update beliefs about the user’s state, which are stored in memory as structured language objects. This belief modeling is a key feature of the Reasoner module, which enables the AI Agent to better understand the user’s needs and intentions and provide more accurate services.
The cooperation between the Talker and Reasoner modules has proven to be effective. The Talker module is able to engage in fluent conversations with users, while the Reasoner module is able to adjust and optimize the tutoring plan based on user feedback. This division of labor allows the AI Agent to simultaneously handle fast-paced conversations and complex planning, greatly improving the user experience.